Credit: Stephen Sauer
Artificial intelligence research is filled with dramatic forecasts. AI will affect almost 40% of jobs around the world, according to the International Monetary Fund. It will increase global GDP by $7 trillion — or 7% — over 10 years, predicts Goldman Sachs. Or it will grow between $17.1 and $25.6 trillion annually, if you prefer to go with McKinsey’s estimate. And these projections are relatively conservative compared with others.
In a new paper, “The Simple Macroeconomics of AI,” MIT Institute Professor has a more conservative estimate of how AI will affect the U.S. economy over the next 10 years. Estimating that only about 5% of tasks will be able to be profitably performed by AI within that time frame, the GDP boost would likely be closer to 1% over that period, Acemoglu suggests. This is a “nontrivial, but modest effect, and certainly much less than both the revolutionary changes some are predicting and the less hyperbolic but still substantial improvements forecast by Goldman Sachs and the McKinsey Global Institute,” he writes.
Acemoglu, a 2024 Nobel laureate in economic sciences, also looked at other economy-wide wage and inequality impacts expected from generative AI.
Deriving a smaller estimate
For the paper, Acemoglu looked at prior scholarship that analyzed which tasks will be exposed to AI and computer vision technologies and concluded that nearly 20% of all tasks in the U.S. labor market could be replaced or augmented by AI. But only about a quarter of those tasks — or 5% economy-wide — could be profitably performed. (In the other 75% of cases, costs for implementation may exceed the benefits.)
Integrating this figure with expectations around productivity — numbers pulled from other scholarship in which researchers studied the deployment of AI in real workplaces — Acemoglu estimates that the total increase in AI-driven productivity over the next 10 years will be roughly 0.7%. This would translate into a maximum growth in GDP of about 1.8%; a more realistic finding under this scenario is about 1.1%.
Daron Acemoglu estimates that the total increase in AI-driven productivity over the next 10 years in the U.S. will be only 0.7%.
But even that is optimistic, Acemoglu writes. So far, generative AI has mostly been used for what he calls “easy-to-learn tasks,” which are defined by two characteristics: There is a straight line between the action and the outcome, and a successful outcome is easy to measure. As AI is incorporated into the economy more broadly, however, it will be applied to a greater number of “hard tasks” — such as diagnosing a persistent cough — and productivity gains will likely be more limited, at least at first.
Acemoglu also notes that there is a mismatch between investment in AI, which is mostly taking place in large companies in certain sectors, and the fact that many tasks that AI can perform or complement are undertaken in small-to-medium-sized enterprises. Finally, as with any technology, there are “adjustment costs” because other aspects of an organization need to evolve and change to function with generative AI. This expense offsets the economic benefits of AI in the near-to-medium term.
Given such caveats, Acemoglu writes, the only change that would make a considerable difference to GDP and productivity estimates would be “to increase the fraction of tasks that will be impacted over the next 10 years” and “to boost the capability of AI models to make new discoveries, such as new materials, new drugs or new services.”
Secondary economic effects of AI
The future value of AI may also be affected by its negative impacts. Acemoglu points specifically to IT and social media — which, despite its ubiquity, can have negative effects on users — as realms where AI may have negative impacts on people’s welfare, through either more powerfully addictive and manipulative algorithms or more sophisticated security threats.
In his research paper, Acemoglu also looks at the potential influence of AI on inequality. Though he doesn’t believe it poses the same threat as earlier types of workforce automation — the effects of which were concentrated in mostly blue-collar jobs — he does foresee it having a negative impact on workers, particularly women with low levels of education.
With these effects in mind, it is possible that GDP will increase while overall welfare declines, Acemoglu writes.
Reorienting the technology
Despite these modest predictions about the economic benefits of AI, Acemoglu said he sees great potential in the technology. But he believes its current trajectory won’t deliver that promise, in large part because it’s being applied to the wrong types of problems in the wrong professions. The focus, he suggests, should be on the provision of truly reliable information within specific problem-solving contexts.
Related Articles
He points to a wide range of roles in which AI use is largely absent, including electrician, plumber, nurse, educator, and clerical worker. People in such professions are typically “engaged in problem-solving tasks,” he writes. “These tasks require real-time, context-dependent and reliable information.”
Think of an electrician who has to deal with an equipment malfunction or a short-circuit on the electricity grid but lacks sufficient knowledge to troubleshoot the problem. If AI tools can provide this kind of information when such workers need it — something the tools aren’t currently reliable enough to do (think hallucinations) — then the technology’s economic impact could be significantly greater, Acemoglu writes.
“There are indeed much bigger gains to be had from generative AI, which is a promising technology, but these gains will remain elusive unless there is a fundamental reorientation of the industry ... in order to focus on reliable information that can increase the marginal productivity of different kinds of workers, rather than prioritizing the development of general human-like conversational tools,” he writes.
“To put it simply, it remains an open question whether we need foundation models … that can engage in human-like conversations and write Shakespearean sonnets if what we want is reliable information useful for educators, healthcare professionals, electricians, plumbers and other craft workers,” Acemoglu writes.
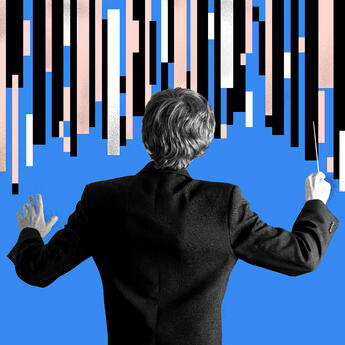
Leading the AI-Driven Organization
In person at MIT Sloan
Register Now