Credit: ChadaYui / iStock
Ideas Made to Matter
It’s time for everyone in your company to understand generative AI
By
A recent survey showed that 70% of marketing companies are using generative artificial intelligence for use cases such as personalization, content creation, and market segmentation, while a 2023 Harris Poll found that about 70% of hiring managers use generative AI. At the same time, about 24% of Americans have used OpenAI’s ChatGPT in their everyday lives, and just 19% think that ChatGPT will have a major impact on their jobs.
As adoption and utilization of generative AI expands, organizations will need to make sure that not only are they using generative AI to the best advantage but that their employees are on board and understand the new technology as well. In a recent webinar hosted by the MIT Sloan Management Review, MIT Sloan senior lecturer and Boston College professor Sam Ransbotham discussed early uses for generative AI, how generative AI compares to previous advanced analytics technology, and why businesses should use caution.
ChatGPT enables anyone to create content
Generative AI tools make it possible for anyone with an internet connection to create customized written, audio, or visual content. When ChatGPT, arguably the most well-known chatbot, was released to the public in November 2022, it became immensely popular, reaching 100 million users in just two months.
One reason for the popularity of generative AI is its widespread applicability, including these use cases for businesses:
- It can generate summaries of lengthy documents or recorded meetings.
- Engineers can give generative AI prompts to write code. This is particularly useful for those working in an unfamiliar language.
- Sales and marketing teams can use it to create highly personalized online shopping experiences by recommending related products.
- Design teams can generate a multitude of models and weed out the impractical ones. This has big potential in automotive design, where brands can spend $3 billion on new models.
- Call centers can provide training powered by conversational assistants, helping new employees get up to speed faster and improve efficiency — and increase the odds that they’ll stay on the job.
As with all AI products, business leaders will want to tread carefully so generative AI will augment human workers and not replace them, Westerman said. “The idea is that generative AI can work with you to make you a better worker,” he said. “It’s a very nice story, as opposed to so many of the stories that are out there about replacing workers.”
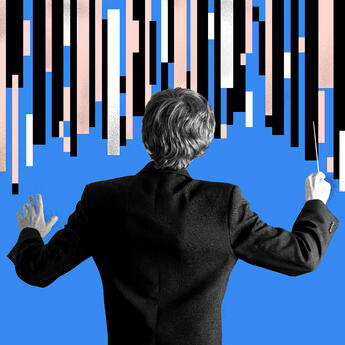
Leading the AI-Driven Organization
In person at MIT Sloan
Register Now
Four types of advanced analytics tools
Generative AI is the latest in a line of advanced analytics tools. The tools vary in how much data and domain expertise is needed to use them, whether their results are repeatable, and how easy it is to understand how they generate results.
Rules-based systems, based on “if-then” automation, have existed for decades, Westerman said. Given their relative simplicity and the limitations of their computing power, this type of AI is typically used with well-understood problems, like processing insurance claims. “You really don’t need a whole lot data, but you do need a lot of expertise,” Westerman said. The results are also highly repeatable and explainable.
Econometrics, the next step forward, estimates relationships between things that can be distilled down to numbers. This requires less expertise than rules-based automation but more data, and, as a result, it requires careful thought about how models will be built. The results are usually repeatable but less explainable than those of rules-based automation.
Deep learning tools enable automated classification of data, process optimization, and predictive modeling. For example, Google used deep learning to reduce the energy consumed to cool its data centers by nearly 40%. “The machines are running the air conditioner better than people are,” Westerman noted. Deep learning requires a lot of data but less domain expertise. The results are somewhat repeatable but not very explainable.
Finally, generative AI enables the automation of creative processes. Like deep learning, generative AI requires little domain expertise but needs lots of data, and it has taken off thanks to accompanying advances in computing power. As Westerman noted, GPT models must work through trillions of data points.
Accuracy, privacy, and other reasons for caution
The power of generative AI also poses concern. Because generative AI tools don’t express their rules or the relationships within their underlying training data, they produce results that are neither repeatable nor explainable. One user’s output might look completely different from another’s, and the outputs aren’t always accurate. If data is incomplete or biased, the results will also be incomplete and biased.
Related Articles
In some ways, this variance is beneficial. When different prompts lead to different results, it can get the creative juices flowing, Westerman said. But if you’re looking to get the same answer every time, generative AI might not be the ideal tool.
All of this should deter business leaders from implementing generative AI hastily, particularly in certain cases.
“Will there be a lot of risk or cost if the model’s wrong?” Westerman asked. “If the financial advice you give can get you sued, or if the medical advice you give can get somebody hurt very badly, then you might want to be careful or put extra controls in place.”
Privacy is another concern. When users type questions into an open generative AI tool, the prompt and the reply become another piece of data for the model to use. Again, this has some benefits — the model has information it can use to continue to learn — but it also introduces the risk that private data will get released to the public or otherwise be used to modify models.
Ultimately, organizations that have seen the most success using generative AI have treated the process like putting a spare tire on a car.
“You don’t screw one bolt in really tightly, because you’re likely to bend things. You get them all on there a little bit, and then you tighten them all up,” Westerman said. “It’s a constant process — do something, do something more, do something more — rather than just trying one technique and sticking with it.”
Read next: Why generative AI needs a creative human touch