Credit: Igor Link / Shutterstock
Ideas Made to Matter
When humans and AI work best together — and when each is better alone
By
One of the most common arguments for bringing artificial intelligence into the enterprise is the potential for AI to help humans by complementing the work they do. But leaders first must understand whether and when AI and humans can perform better together than either can on its own.
A recent paper from researchers at the MIT Center for Collective Intelligence found that on average, AI-human combinations do not outperform the best human-only or AI-only system.
“This was our most surprising finding,” said MIT Sloan professor CCI’s director. “Some of the most important and interesting use cases for AI involve a combination of humans and computers. Many people would have assumed the combination would be quite a bit better, but it was statistically significantly worse.”
The paper, based on a review of more than 100 studies on human-AI collaboration, was published in the journal Nature Human Behaviour. The research sheds light on when the combination of AI and human workers is most poised to succeed — such as tasks in which humans outperform AI on its own, tasks involving creating content, and creation tasks involving generative AI.
Combinations work when humans, AI do what they do best
Malone and his co-authors — MIT Sloan assistant professor and Michelle Vaccaro, an MIT doctoral student and CCI affiliate — analyzed 370 unique effect sizes from 106 experiments that evaluated the performance of humans alone, AI alone, and human-AI combinations. The studies were published between January 2020 and July 2023. (Effect size is defined as the magnitude of the difference between variables in a study.)
The researchers found that the combination of humans and AI outperformed the baseline of humans acting on their own, but it did not perform better than the baseline of AI on its own. Notably, the average performance scores for the combination of humans and AI were lower than those of the best human or AI systems.
For example, AI alone proved proved to be the most success at detecting fake hotel reviews, with an accuracy rate of 73%, compared with 69% for humans and AI together and 55% for humans alone. The researchers hypothesized that because people were less accurate at the task in general than the AI, they were also not very good at deciding when to trust the algorithms and when to trust their own judgment. This resulted in lower performance for the combination of AI and humans than for AI alone.
“Combinations of humans and AI work best when each party can do the thing they do better than the other,” Malone said.
Other examples of AI outperforming humans and the AI-human combination include forecasting demand and diagnosing medical issues.
In scenarios where humans performed better, the humans and AI working together outperformed either alone, on average. Take, for example, classifying images of birds — a task that requires specialized expertise. Humans alone achieved 81% accuracy, and AI alone achieved 73% accuracy, but the combination hit 90% accuracy.
“If a human alone is better, then the human is probably better than AI at knowing when to trust the AI and when to trust the human.” Malone said.
Redefining processes is better than reassigning tasks
The researchers said human-AI collaboration can take two different forms. Human-AI augmentation takes place when the average human-AI system performs better than a human alone. Human-AI synergy occurs when human-AI output outperforms both humans and AI alone.
Achieving human-AI synergy is hindered by several challenges. The first is understanding when humans alone, AI alone, or the combination of the two will be most effective. Many organizations struggle with this, Vaccaro said, because they tend to overestimate the effectiveness of the systems they have in place. Randomized experiments, such as A/B tests that evaluate outcomes across the three use cases, can provide data-driven insights here.
The second is applying the results of such experiments to achieve change. This is less about dividing subtasks between humans and AI, Malone said, and more about redesigning the whole process of how they work together. Companies looking to automate the mass production of furniture, for example, would need to consider whether they should automate not just the intricate steps of assembly but also the onerous process of moving a finished wardrobe across the factory floor.
“We found humans excel at subtasks involving contextual understanding and emotional intelligence, while AI systems excel at subtasks that are repetitive, high-volume, or data-driven,” Vaccaro said.
After deciding on a strategy, it pays to adopt a model of continuous improvement. “Start with a basic workflow, then monitor performance, and, finally, refine the workflow based on outcomes and user feedback,” she said.
Generative AI shows the power of collaboration
One area of promising synergy between humans and machines is generative AI.
The researchers found that human-AI combinations performed worse on tasks that involved decision-making but better on tasks that involved creating content. Creation tasks were relatively unexplored during the study period — only 10% of papers that were reviewed looked at content creation. But in those cases, “the average effect size for human-AI synergy was positive and significantly greater than that for the decision[-making] tasks,” which made up the bulk of the research and tended to have negative effects, the researchers write.
Related Articles
In a previous paper, Malone and his coauthors explored how generative AI can inspire creative workers by generating a multitude of images from a simple text prompt in a matter of seconds. Although some results may be silly and irrelevant, the process is far faster than a designer with a sketchpad — and it can provide inspiration for more complex designs that would benefit from the human touch.
Vaccaro said the iterative loop that’s possible with generative AI makes it better suited for human collaboration than earlier AI systems designed primarily to complete specific tasks.
“Generative AI systems allow for a more iterative and interactive process,” she said. “Humans can now collaborate with generative AI in a cycle of drafting, editing, and reworking text, images, music, or videos. The AI can adapt to human feedback in real time, which enables humans to refine their outputs dynamically.”
The paper’s conclusion that the combination of humans and AI may not outperform humans and AI alone may give enterprises reason to pause their efforts at collaboration. But Malone said that’s the wrong lesson to draw, in part because of generative AI’s promising capabilities.
“What we’re saying is that we need to become more sophisticated and knowledgeable about what works for human-AI collaboration and what doesn’t,” he said.
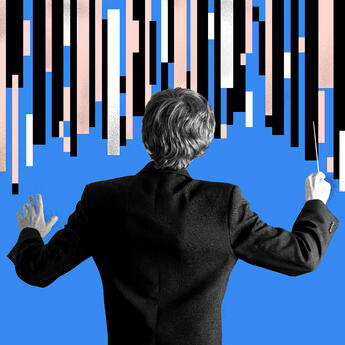
Leading the AI-Driven Organization
In person at MIT Sloan
Register Now